Computer scientists at MIT join forces with oceanographers to develop a machine-learning model that accurately predicts ocean currents.
- May 23, 2023
- Posted by: Sinead Sprigg
- Category: Engineering, Environmental, Technology & Innovation, North America
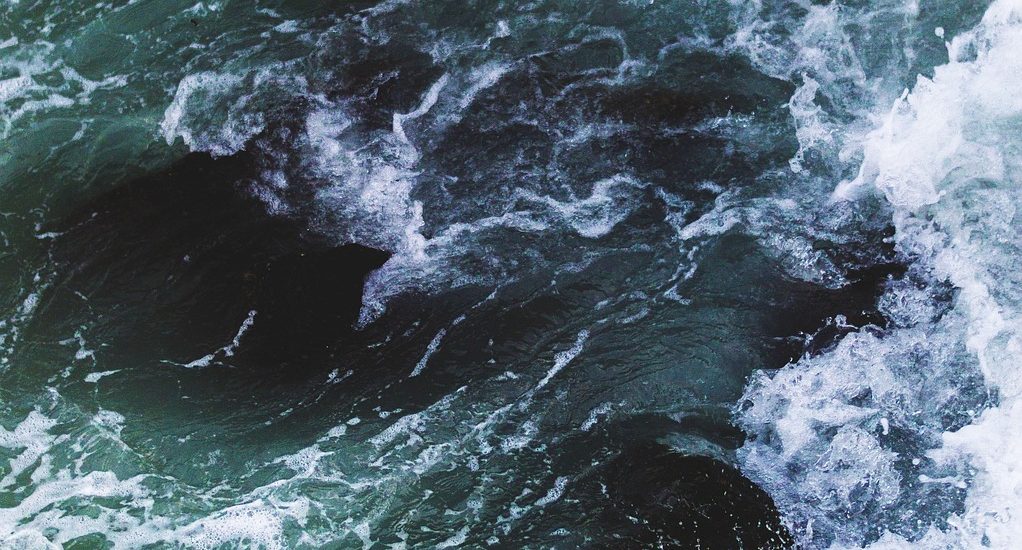
Scientists have developed a new model that incorporates machine learning to improve the study of ocean currents. Currently, researchers release GPS-tagged buoys in the ocean and record their velocities to understand the currents that carry them. These buoy data are also used to identify areas of divergence, where water rises or sinks below the surface. By accurately predicting currents and pinpointing divergences, scientists can enhance weather forecasting, estimate the spread of oil spills, measure energy transfer in the ocean, and monitor the transportation of various substances.
The multidisciplinary research team, including computer scientists at MIT and oceanographers, discovered that the conventional statistical model used on buoy data often struggles to accurately reconstruct currents or identify divergences due to unrealistic assumptions about water behavior. To address this issue, they developed a new model that incorporates knowledge from fluid dynamics to better reflect the physics involved in ocean currents. The researchers demonstrated that their method, which involves a small additional computational expense, provides more accurate predictions of currents and identification of divergences compared to the traditional model.
The new model has the potential to enable oceanographers to make more accurate estimates from buoy data, allowing for more effective monitoring of the transportation of various substances such as biomass, carbon, plastics, oil, and nutrients in the ocean. This information is crucial for understanding climate change and tracking its effects. The researchers emphasized that their model captures the physical assumptions more appropriately, leveraging existing knowledge of ocean physics to focus on learning important aspects such as currents away from the buoys and the occurrence and location of divergences.
The model utilizes a Helmholtz decomposition, a method from fluid dynamics, to accurately represent the principles of fluid motion. By breaking down an ocean current into vorticity (capturing whirling motion) and divergence (capturing rising or sinking water), the model incorporates basic physics knowledge to improve predictions. The researchers evaluated the model using both synthetic and real ocean buoy data, demonstrating its superior performance in predicting currents and identifying divergences compared to standard Gaussian process models and a neural network approach.
In future work, the researchers plan to incorporate a time element into their model to account for variations in currents over time. They also aim to better account for noise in the data, such as the influence of winds on buoy velocity, which could further enhance the model’s accuracy. The research team believes that their approach will enable the prediction of divergence and vorticity away from the buoys based on noisily observed velocities, leading to improved understanding of ocean dynamics.
Experts not involved in the research have praised the integration of known behaviors from fluid dynamics into the model, highlighting its flexibility and ability to capture phenomena such as vortices and connected currents. The research is supported by the Office of Naval Research and the National Science Foundation, among others.